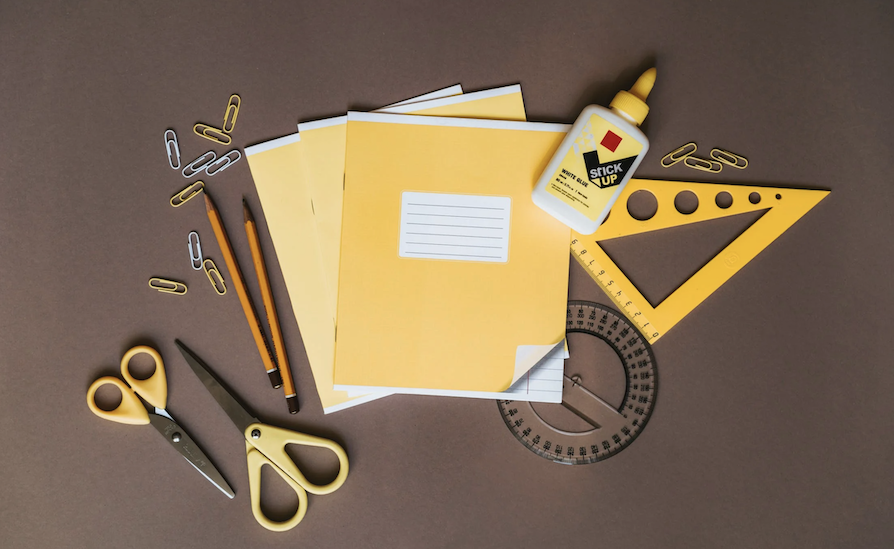
We make sense of the world through models we create in our minds. How well we understand reality depends on the accuracy of those models. However, we are often not aware that our internal representation can be inaccurate or, worse, we may not even realise that we use mental models at all. By bringing our thought processes into the open, we can shed light on the gaps in our understanding and learn more efficiently.
I have written about the role of mental models in software engineering. I recommend reading that first if you haven’t done so, as it explains the main idea. In this post (and more to follow), I take an existing tool, framework or subject and look at it through the lenses of mental models.
Today’s example is Git. It assumes little or no prior knowledge. If you are already well-versed in Git, I encourage you to keep reading nonetheless. The goal of this series is as much teaching a specific subject as it is about exploring how to construct good mental models in general. There is no shortcut to achieving accurate understanding. As good as an existing mental model may be, we still have to absorb and make it our own; we can’t just copy and paste it into our brains (unfortunately).
What is Git?
The official definition says this:
Git is a distributed version control system.
Does reading this sentence evoke any mental images for you? In my case, the word “distributed” brings to mind several computers running in distant locations. “Version control system” lets me see software that stores copies of documents in an orderly fashion.
This is rather vague. A better way to understand something is not to ask “what” it is, but “why” does it exist - what is its purpose?
What is the purpose of Git?
There are primarily two problems Git is trying to solve:
- How can multiple people work in parallel on the same documents without stepping on each other’s toes?
- How can we provide a safe environment for making changes without worrying about losing previous work?
2) is part of the solution for 1). However, it is also possible to only take advantage of 2) while ignoring 1) (if you use Git solely as a local version tracker, which is a legitimate use case).
1 - How can multiple people work on the same documents at the same time?
Let’s think about 1) - there is a group of people who want to work on the same set of documents simultaneously. How can they do that? Pose that question to yourself, and your brain will immediately start generating possible answers.
Depending on how much experience you have with a given problem, the solution you come up with can be complex or straightforward. A natural way to find a solution to a problem is to search in our memory for a similar situation that we can apply to the new challenge. How do people typically collaborate?
I see an office where several people are sitting around a table, with paper documents spread out in front of them. They pass files around, discuss changes, use pencil and eraser (or scissors and tape) to make changes.
Something like this:
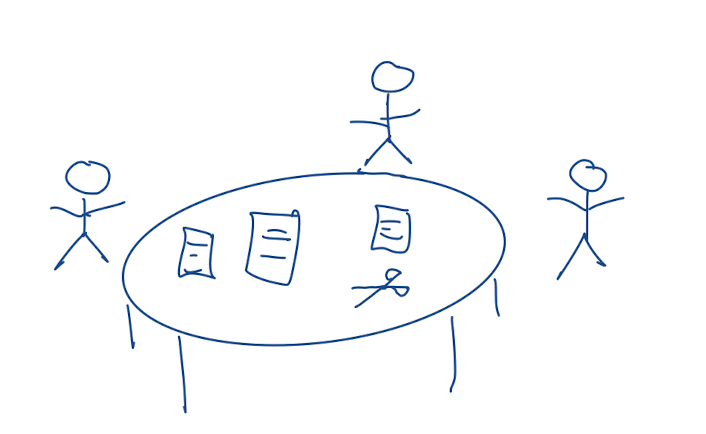
Although this model describes collaboration, the participants can’t work in parallel, as they need to take turns to change a document. Let’s refine our model by having everyone work on copies instead of the original documents. To reflect that Git is a distributed system, we also let each worker have their own table.
Our new model looks like this:
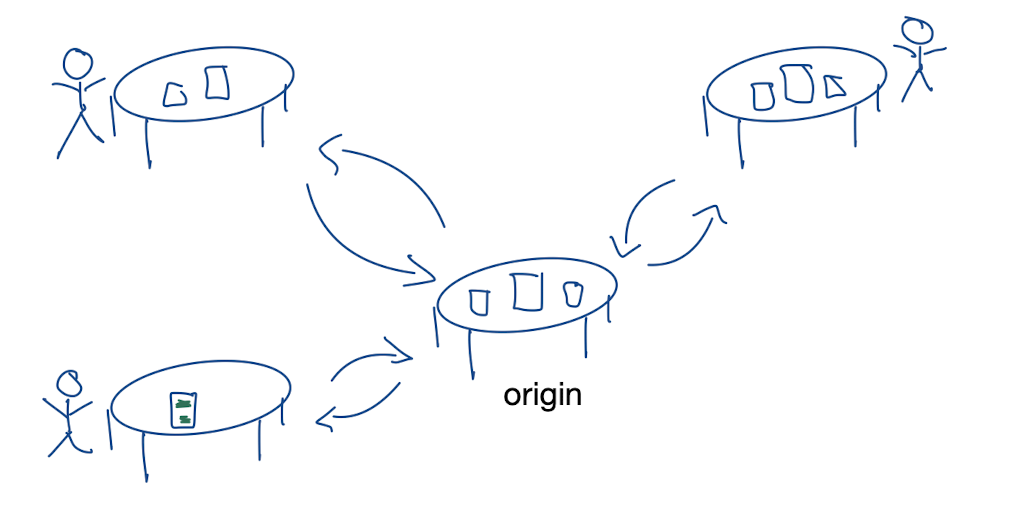
How would that work? The table in the middle (let’s call it the “origin” table) contains the original documents. If I want to work on them, I first make copies of the documents and then grab one of the tables and go about making changes as I please. Then comes the moment to update the originals. I go back to the origin table and replace the sources with my edited copies.
There’s just one problem: what if someone else has already changed the originals? If I swap the documents, their changes will get lost.
So instead of swapping out entire documents, I need to “merge” my changes with whatever is on the origin table. This works most of the time, except when my change and someone else’s change are in “conflict”. For example, in a design document, someone changed the color of a button from red to green, but I changed it from red to yellow. Who’s change should persist?
A conflict:
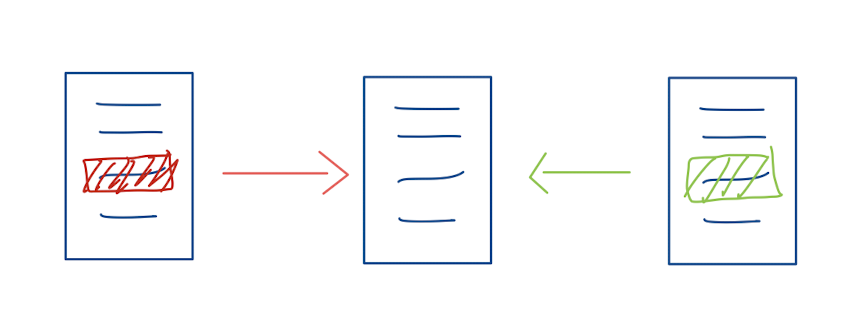
I want to keep my version, but what if I change my mind later (because it turns out my colleague was right)? That is the second problem Git solves: as long as we track changes and can revert back to a previous state, we are safe to make changes.
How does Git make that possible?
Assume I have no spontaneous idea. We are then at a typical state during the learning process. We have exhausted fleshing out our mental model (or don’t want to speculate anymore), and we encountered a few areas where we can’t satisfyingly figure out how things work in reality.
This is a good moment to do some research and consult a book or a manual.
Sidenote: why start with the mental model?
You may ask why we didn’t start reading the book before building our model. Aren’t we wasting our time trying to come up with an explanation of how things work without having done research first? I disagree. By having made our current knowledge visible, the subsequent research becomes more targeted. We can locate gaps more quickly, know what kind of information we need or don’t need, and have a map to place new information.
2 - Keeping a log of changes
So how does Git keep a log of changes?
I use Pro Git as reference documentation. A good chapter to start is What is Git?, you may want to read it now.
The main point from that chapter leads us to the answer to our question:
Git maintains snapshots of changes, not diffs
In other words, every change in Git is stored as a snapshot of all files. Note that the word “snapshot” is another mental construct. Like a camera that catches a scene at a specific moment, a snapshot in computing is a copy of an entire state of a system at a certain time. We can use that information to extend our mental model.
Besides working on our copies of the original documents,
we create additional copies of the copies and put them in boxes. Each box reflects a document change. To keep a log of changes, each box links to the previous box.
It looks like this:
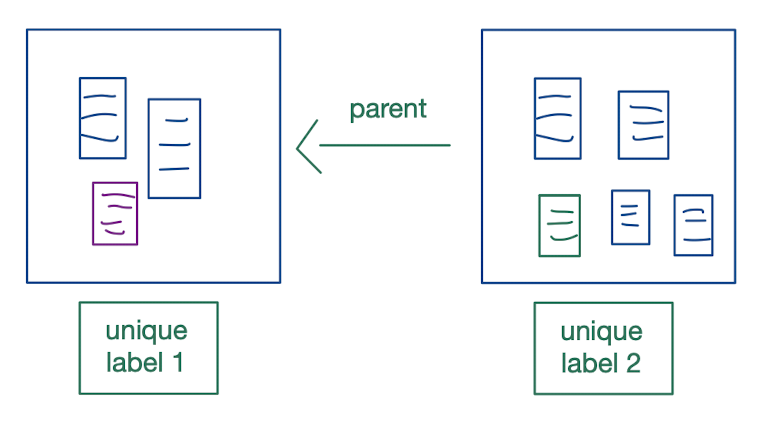
Those boxes are uniquely labelled. As you may have read in the book, Git creates so-called hashes of documents (you’ll find hashes everywhere in Git). A hash is a string of symbols that is unique for any possible state of documents.
You may want to dig deeper to understand how hashing works under the hood. Or not? That is another common stage of any learning process. The question is this: should I figure out the inner workings of hashes, or should I just accept that they work in some way and move on?
Your mental model can guide you here as well. At this point, knowing how hashes work won’t help you with your understanding of Git. It won’t increase the accuracy of your model at this stage (being a beginner). It is the wrong rabbit hole to go down. Instead, for the sake of simplicity, we accept this gap in our understanding.
Good mental models strive for simplicity by conveying just enough details that are required to reliably predict the behaviour of the thing you are investigating. Any additional information will slow down progress (more about this at the end).
Log a change - but when?
Back to Git. Another question remains: when do we take a snapshot? Could we store every change? That would create an insurmountable amount of data and not be feasible.
Instead, what seems practical is that the author decides when to store a snapshot. Only they know when changes have reached a meaningful state that should be preserved.
How does Git make that possible?
The same Pro Git chapter mentioned above answers that question. The relevant paragraph is called “the three states”.
Three states
A file in a Git repository can have three different states: it is either “untracked”, “staged”, or “committed”. Untracked files (or modifications) are not recorded yet. When files are “staged”, that’s the moment when we intend to take a snapshot (but we haven’t registered the change yet). Committing files is the final step of storing the snapshot.
The three states:
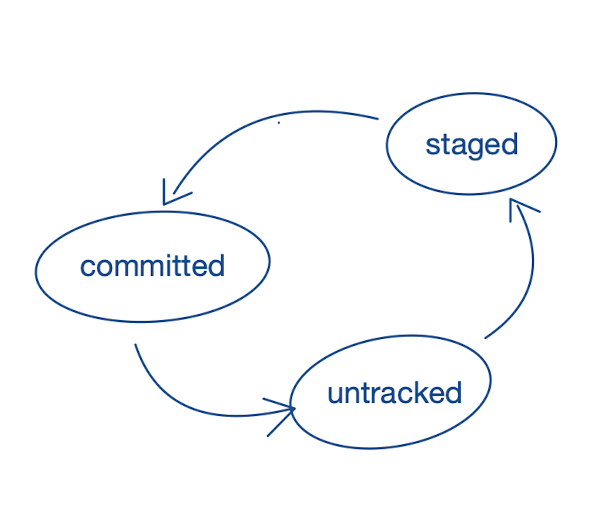
Coming back to the question of when to take a snapshot, the answer is that it is up to the user to make that decision.
By the way, Git introduces three states, although two would have been sufficient (e.g. committed - or not committed). The intermediate staging step makes preparing a snapshot easier, as we can add or remove files and change our minds before creating the record.
Testing our model
We have developed a set of mental models that cover the basics of Git. It is time to test the usefulness of those models by asking ourselves if they can sufficiently explain how things work for our purposes.
Branching
What is a branch? The word is already evoking a mental model of a real tree, with its main trunk and branches diverging into new directions.
You can read in detail about Git branches in Pro Git, but we can already explain how branches work in principle. As discussed above, “how Git creates a log of changes”, a branch is nothing other than a series of snapshots that is different from the main development flow. Internally, Git uses pointers to make that work, but all we need to understand now is that changes are stored as snapshots and that each snapshot has a unique label (hash) attached to it. If you want to know if two branches are the same, all you have to do is compare the hashes.
Git commands
We test our model by looking at basic Git commands that you will likely have encountered when you first learned about Git.
git clone
git add
git commit
git push
git pull
If you are new to Git, I recommend running git COMMAND --help
and start reading through the first paragraphs to see if the explanation fits our mental models.
git clone --help
says
Clones a repository into a newly-created directory, creates remote-tracking branches for each branch in the cloned repository (visible using git branch –remotes), and creates and checks out an initial branch that is forked from the cloned repository’s currently active branch.
We may not fully understand every detail, but the overall idea fits our distributed tables model of making copies and branches.
git add --help
This command updates the index using the current content found in the working tree, to prepare the content staged for the next commit.
We don’t know yet what an index is but can explain most of what is said here with the three stages model. And we learn that git add
moves changes from “untracked” to “staged”.
git commit --help
Create a new commit containing the current contents of the index and the given log message describing the changes.
Same here, and now we can conclude that the “index” tracks changes that have been “staged”.
git push --help
Updates remote refs using local refs, while sending objects necessary to complete the given refs.
We don’t know what a ref is but “sending objects” fits our model where we bring our documents to the “origin” table and merge our changes.
Incorporates changes from a remote repository into the current branch. In its default mode, git pull is shorthand for Git fetch followed by Git merge FETCH_HEAD.
This does not fit our mental model. So far, we said that when we are ready with our work, we bring our changes to the “origin” table. But in reality, we “pull” changes down to us. We found a gap in our model. Coming back to Pro git - What is git? we can now understand what is meant by Nearly Every Operation Is Local. If Git was new to you and you followed along with the steps of this article, I bet you have now a stronger understanding of what “everything is local” means compared to when you first time read about it.
“Real-life” testing
The ultimate test occurs when we apply our knowledge to real-life situations. Whenever Git does not behave as expected, we find out where we need to improve the model.
Polish & refine
As mentioned in the introduction post, building mental models is a four-step process:
- Define your model. We all use some kind of model, whether we are aware of it or not.
- Test your model. Either by researching or in real-life situations.
- Improve your model. Take what you have learned in 2) and improve it. Then test again.
- Simplify or go deeper. There will come a moment when steps 2) and 3) won’t bring any further improvements or when the model does not go deep enough because you have advanced in your understanding and tackling bigger challenges. You may be at a point where you can significantly simplify the model or build a new one.
We have not yet exhausted steps 2) and 3), but we can still simplify our model. The table metaphor has gaps and is not that useful (but it helped to develop the model of snapshots).
Instead, the model of snapshots and the three file states together seem to be a good first mental model of Git.
The final Git mental model
Log of hashes & three states
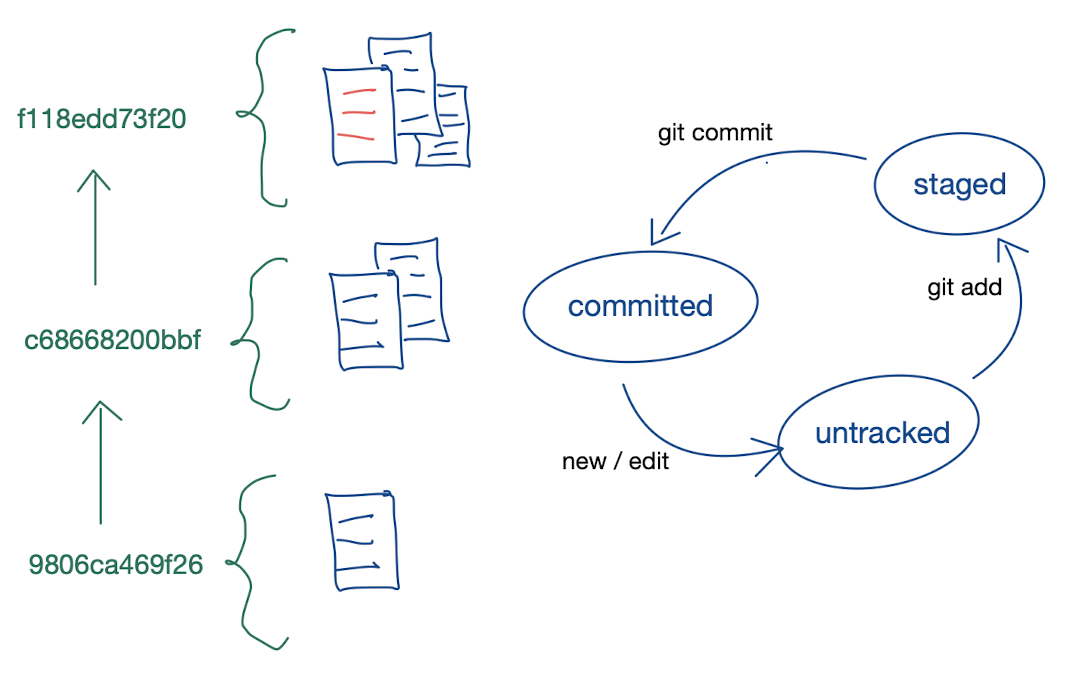
- Every committed change in Git is stored as a snapshot and referenced by a unique hash. A log of changes is represented by a log of hashes. To compare two branches, all we need to do is compare the hashes to know if they are the same or not.
- All files go through three possible states.
Summary
While developing an understanding of how Git works, we have gone through the process of constructing mental models. The key takeaways are the following:
Always build your mental model first. Before you start reading a book or tutorial, sit down and visualise how you believe that the thing you are interested in works. That will make your subsequent research a lot more efficient, as you have a base that guides your reading and a place where you can place new information and relate it to existing knowledge. Remember the last time you read a book without any preparation. You may have highlighted sentences and written some notes in the margins - but how much of that information could you actually remember?
Test and refine your model. That step is easy to forget, especially when it comes to improving your mental model. When you hit a plateau in your learning effort, that is the moment where you need to go back to your model and work out where the gaps are.
Just enough information Good mental models are simple, some even elegant, and they convey just enough information to be useful.
The goal is not 100% correctness. A model is always an approximation and depends on your context and level of understanding. Don’t try to cram everything in it. Instead, figure out what are the most important facts of something. That exercise itself is very valuable to master a skill.
Next
With the model at hand, we can now go and apply it to our daily work.
A good idea might be to look at your .git
folder and try to figure out what is going on there. That will give you a deeper understanding of the internals (the relevant chapter in Pro Git is Git Internals Plumbing and Porcelain).
Or even better, don’t do anything and just use Git and wait until you hit a bump. That is the beauty of learning with mental models. There is no fixed path that you have to follow - real-life situations will show you where you need to improve.
I will come back to Git in a follow-up post and apply the model to more advanced situations, showcasing examples of where our model is sufficient and where it is not.